Abstract
Background: Prior research confirmed that the balanced scorecard (BSC) can be used successfully at manufacturing small and medium enterprises (SMEs), to assist with sustainability. South African SMEs have a low survival rate despite being a significant contributor to the local economy with the manufacturing sector in particular hampered by negative growth.
Aim: The objective of this study was to develop a BSC for manufacturing SMEs in South Africa with measurable key performance indicators (KPIs).
Setting: We conducted a Delphi study with cost accounting specialists in different industries.
Methods: The development of the generic BSC was facilitated with a Delphi survey and analytical hierarchy process (AHP).
Results: The research presents a generic yet flexible BSC for manufacturing SMEs. A total number of 12 generic and 104 specific KPIs were identified. The results revealed a greater emphasis on the financial and customer perspectives that may be conducive to SME sustainability and success.
Conclusion: A generic BSC that can be adapted to specific organisational and industry requirements has the potential to enhance SME sustainability and success.
Introduction
South African small and medium enterprises (SMEs) contribute up to 22% of gross domestic product in the economy (Bureau for Economic Research 2016:31). Yet the survival rate of South African SMEs is very low, with nearly 80% of all SMEs failing over the long term (Brink, Cant & Ligthelm 2003:1; Olawale & Garwe 2010:729). The manufacturing sector is particularly vulnerable because of higher labour costs in comparison to other sectors, which results in a declined prevalence of manufacturing SMEs (Bureau for Economic Research 2016:20). The high labour costs are further exacerbated by South African labour laws, which render the lay-off of unproductive and redundant staff cumbersome at best (Bureau for Economic Research 2016:8). In addition, the high crime rate in South Africa could hamper the development of manufacturing SMEs, probably as a result of increased security costs (Sesep 2016:44). Difficulties obtaining financing and inexperienced entrepreneurs are additional contributing factors to the demise of manufacturing SMEs (Brink et al. 2003:18; Olawale & Garwe 2010:735). To facilitate the development of SMEs in South Africa, the National Development Plan (NDP) was introduced by the South African National Planning Commission (Ingle 2014:37). Ingle opines that, although the NDP acknowledges the high labour costs and social problems, there may be other factors limiting the growth of SMEs (Ingle 2014:38).
As an important contributor to the South African economy, how can SMEs’ sustainability be improved? The balanced scorecard (BSC) is a measurement tool that may be used by an organisation to measure its financial and non-financial performance (Kaplan & Norton 1992:71). The BSC could enable an organisation to achieve its long-term strategic goals by managing the short-term targets (Okongwu, Brulhart & Moncef 2015:698). Furthermore, it may allow organisations to focus their attention only on those activities that are beneficial to the achievement of its strategic goals (Andersen, Cobbold & Lawrie 2001:7). As a result, the BSC is considered a useful management tool for SMEs, although its implementation may differ from that of larger organisations (Andersen et al. 2001:9; Fernandes, Raja & Whalley 2006:633).
Critical success factors (CSFs) are those factors contributing to an organisation’s long-term survival (Rockart 1979:85). Extant literature identifies different CSFs for SMEs in developing countries. Two primary CSFs associated with SMEs in developing countries are operating costs and access to financial resources (Nuntsu, Tassiopoulos & Haydam 2004:521; Sesep 2016:57). It is considered important for SMEs to identify and address their CSFs to ensure sustained growth (Brink et al. 2003:19; Ng & Kee 2012:685; Temtime & Pansiri 2004:19). In order to address these CSFs, the BSC can thus be useful to owners and managers of SMEs (Ayvaz & Pehlivanl 2011:146), because the BSC aligns the organisation with its strategic goals (Andersen et al. 2001:9). Measurements that can be quantified and used to track the CSFs of an organisation, are key performance indicators (KPIs) (Fernandes et al. 2006:624). Fernandes et al. (2006:631) suggest that SMEs should only focus on the essential KPIs when implementing the BSC, that is on the quality of KPIs rather than increasing the number of KPIs. It is thus imperative that CSFs for SMEs be investigated.
It is evident from the literature consulted that the high failure rate of SMEs in South Africa has an adverse effect on economic growth (Bureau for Economic Research 2016; Ingle 2014; Sesep 2016). This may partly be attributable to two key aspects. Firstly, the generic CSFs for the sustained survival of manufacturing SMEs in South Africa are not clearly outlined in published literature; and secondly, it is not clear how these CSFs should be considered in a generic BSC for manufacturing SMEs’ sustainability.
The research objective of this article is to propose measurable KPIs that should be considered in a generic BSC for manufacturing SMEs. In doing so, this article defines the context of the manufacturing SME and its generic functions; identifies the CSFs necessary for manufacturing SMEs to gain a competitive advantage; determines how the CSFs can be considered in the BSC; and outlines the KPIs to be included in the generic BSC for manufacturing SMEs.
The manufacturing small or medium enterprise and generic critical success factors
The modern manufacturing industry likely originated during the British Industrial Revolution in the 18th century as described by Kelly, Mokyr and O’Grada (2014). The process of manufacturing includes people, machinery and tools in a facility such as a factory to produce a product for a customer (Obi 2013:3–4; Rajput 2007:1). Manufacturing organisations in the SME category in South Africa are defined as manufacturing organisations with an annual turnover of less than R163 million, with some factories having a turnover of less than R2 million (Statistics South Africa 2017:30).
Literature revealed that six generic functions or activities (or departments) could be identified at a manufacturing SME, namely production and product development; sales and distribution; customer service; purchasing; marketing; and management and administration (Jespersen & Skjøtt-Larsen 2005:18; Kahn 2015:46; Obi 2013:12). It is likely that CSFs can be attributed to specific activities. Production was found to be essential and useful to measure performance (Bhagwat & Sharma 2007:48; Gunasekaran, Patel & McGaughey 2004:337; Khan & Tidke 2013:1; Kumar et al. 2016:1300). Product development is considered an important function because of its focus on innovation and reduction of costs (Dhurup & Makhitha 2014:232; Mendis & Ganga 2013:93). The supply chain management (SCM) function consists of the sales and distribution function, customer service and the purchasing function (Jespersen & Skjøtt-Larsen 2005:13). Measuring the performance of the SCM functions allows managers to direct their focus at areas of improvement (Afonso & Cabrita 2015:279; Callado & Jack 2015:288; Okongwu et al. 2015:698). Measuring the CSFs within the BSC could improve the effectiveness of the marketing function (Engle 2005:135), which may have a significant influence on the overall performance of a manufacturing SME (Mokhtar, Yusoff & Ahmad 2009:80; Mokhtar, Yusoff & Arshad 2014:57). It is plausible that measuring the six activities within the BSC could enhance the effectiveness of the BSC. The performance of the six activities incorporated in a BSC is affected by the CSFs, which is addressed next.
Owners of SMEs in South Africa often have limited business acumen and the potential failure of SMEs can likely be attributed to this lack of skill (Kirsten, Vermaak & Wolmarans 2015:32). The competence of the owner and manager of the manufacturing SME can be considered as a CSF necessary for its sustainability (Asare et al. 2015:32; Nkosi, Bounds & Goldman 2013:9; Okpara & Kabongo 2009:16; Okpara & Wynn 2007:33). It can thus be argued that the performance measurement of the management and administration function is important for manufacturing SMEs’ sustainability.
Another CSF at manufacturing SMEs that may be of importance to ensure suppliers are paid and production lines are running, is cash flow management (Sebone & Barry 2009:193). A lack of cash flow may be attributed to the lack of access to financing, which is a common barrier for manufacturing SMEs (Asare et al. 2015:32; Ghosh et al. 2001:209; Moyo 2003:169; Okpara & Kabongo 2009:15; Okpara & Wynn 2007:31; Yusuf 1995:72). Because of the challenge of obtaining low-cost loans, SMEs are left with no choice but to opt for more expensive financing options (Okpara & Wynn 2007:31). The relationship between cash flow and the cost of financing suggests that measurement within a BSC may have to be conducted in parallel.
Government support can also be regarded as necessary for the sustainability of manufacturing SMEs, because a lack of government support could contribute to failure to increase their revenues (Moyo 2003:169; Onaolapo & Oladejo 2011:318). Failure to increase revenue because of a lack of government support can likely be attributed to a lack of funding to grow the customer base of manufacturing SMEs. Apart from increasing revenues, manufacturing SMEs can also reduce operating costs to increase profits (Hung, Hung & Lin 2015:200). This suggests that owners could counter a limited revenue base by managing their operating cost. The measurement of revenue, as well as cost, could therefore be regarded as generic CSFs for manufacturing SMEs.
To produce a high-quality product, it is vital to ensure the basic elements of total quality management (TQM) (Charantimath 2011:76) are adhered to. Measuring the effectiveness of TQM can enhance the financial performance of an organisation (Mehralian et al. 2017:120). A high-quality product may form a basis for fostering customer relationships that is considered essential for organisational success (Ghosh et al. 2001:209; Moyo 2003:168). The customer relationship of the manufacturing SME needs to be maintained by a good product and at a competitive price, as well as with an effective aftersales service (Benzing, Chu & Kara 2009:63; Ghosh et al. 2001:211; Temtime & Pansiri 2004:23). Customer relationships can also be influenced by the on-time delivery performance of the SME (Belekoukias, Garza-Reyes & Kumar 2014:5361; Hung et al. 2015:198). There may also be a relationship between customer service delivery and the effectiveness of TQM (Mehralian et al. 2017:120). It is plausible that customer requirements such as value for money, quality and acceptable service delivery have to be monitored from the customer’s viewpoint and internally to ensure that the cause and effect of these measures are addressed. These customer requirements could, therefore, be regarded as CSFs for manufacturing SMEs.
There may be an argument that resources such as ‘people and machinery necessary for customer satisfaction’ and ‘internal management’ must be managed and monitored. The development of people in the workplace was shown to be a CSF for SMEs (Avcikurt, Altay & Ilban 2011:161; Sebone & Barry 2009:192). By training people, it is possible to improve labour productivity, which is considered vital for manufacturing SME success (Santos-Requejo & González-Benito 2000:216). Labour productivity, customer satisfaction and the organisational performance, in general, can also be improved if employees are satisfied in the working environment (Antoncic & Antoncic 2011:600; Jeon & Choi 2012:341). It is likely that non-measurement of people and machinery could have an impact on the CSFs mentioned earlier and it may, therefore, have to be considered as a generic CSF for manufacturing SMEs.
Information and communications technology (ICT) is another critical contributing factor to manufacturing SMEs’ success. The effective implementation and use of ICT at manufacturing SMEs can influence the financial success and market growth of the organisation (Dhurup & Makhitha 2014:246; Gono, Harindranath & Özcan 2014:14). In addition to ICT, the status and relevance of production technology may also be necessary for manufacturing SMEs to prosper (Santos-Requejo & González-Benito 2000:215).
Balanced scorecard and development techniques
As mentioned earlier, the BSC is used by organisations to review non-financial and financial measures (Kaplan & Norton 1992:71). The BSC consists of four perspectives (Kaplan & Norton 1992:71), namely financial, customer, internal, as well as learning and growth, in which several metrics could be evaluated. The financial perspective represents metrics from the shareholders’ point of view and is typically measured in monetary terms. The customer perspective relates to the metrics that could indicate in what manner customer requirements are satisfied. The internal perspective provides an overview of the metrics that evaluate the internal performance of an organisation. The learning and growth perspective reviews the metrics that measure internal growth and development. Lin (2015:1239) has suggested that there is a relationship between the results from the non-financial perspectives and profitability. The development of a generic BSC could be an effective tool for manufacturing SMEs to increase profits by managing the important metrics.
The development of the BSC is often used in conjunction with Delphi studies and the analytical hierarchy process (AHP). Delphi studies, using multiple surveys, are conducted when consensus is required on specific elements (Hasson, Keeney & McKenna 2000:1008; Remenyi 2013:70). A panel of experts is assembled to conduct a Delphi study (Shelton & Creghan 2015:376). The AHP is used for decision making and involves the use of pairwise comparisons (Saaty 2008:85). It is suggested that the AHP allows the relative importance of BSC perspectives and metrics to be established (Varma, Wadhwa & Deshmukh 2008:353). A scale of 1–9 is typically used to compare the elements (Table 1).
TABLE 1: Scale of numbers for analytical hierarchy process elements. |
Once a problem is identified for which the AHP can be used, it is necessary to create a hierarchy design (Saaty 2008:85). As an example, in the study of Ahammed and Azeem (2013:6–11), it was required to establish the most suitable solar power system for a rural area. The solar power systems had different power outputs (75 Wp, 50 Wp, 30 Wp), and each of the solar power systems had relative positives with respect to cost, ability and availability (Figure 1). These attributes were considered for the decision criteria.
 |
FIGURE 1: Hierarchy design for decision making. |
|
After the pairwise comparisons are conducted, it is possible to commence the AHP analysis. The first step is to calculate the relative weights for each solar power alternative (Ax). A comparison matrix (Eqn 1) is created for the relative weights. In addition, a relative weight calculation into a normalised matrix (Eqn 2) is conducted for each Ax by dividing column elements with column averages (Ahammed & Azeem 2013:8).
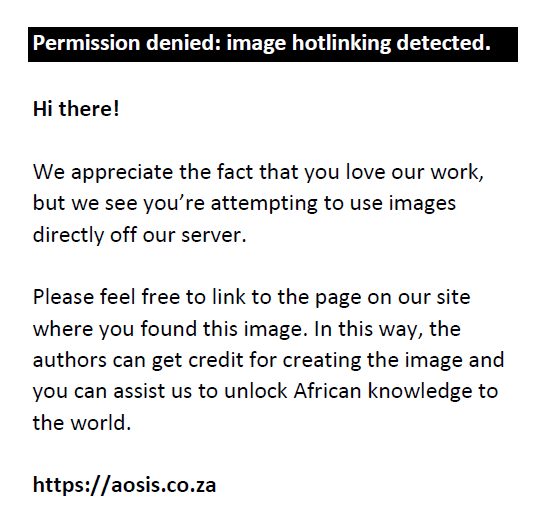
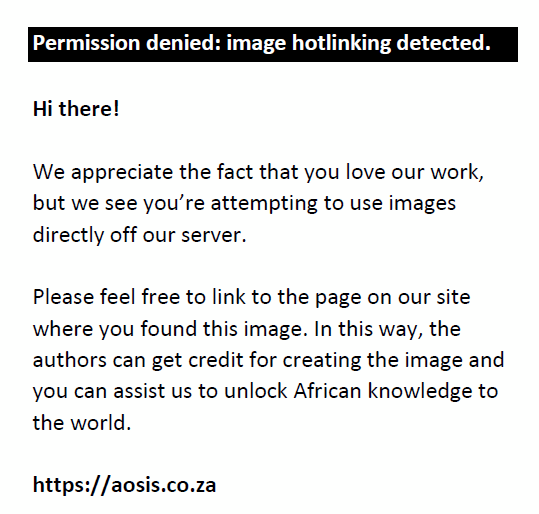
According to Mu and Pereyra-Rojas (2017:11), the activity of normalising the comparison matrix (relative weight calculation) refers to the approximate AHP method, a simpler form of AHP. The overall priorities as selected by a decision-maker can be calculated by using the average of each row in the normalised matrix. A consideration for pairwise comparisons is the consistency of the selections made by expert panels. The expectation is that selections are reasonably consistent and that perfect consistency is not normal (Ahammed & Azeem 2013:8). Consistency in selections in the AHP process is measured by calculating a consistency index (CI / Eqn 3) and a consistency ratio (CR / Eqn 4). The variable λmax is calculated by using the priorities calculated for each row and multiplied with the comparison matrix. To calculate λmax the weighted total for each row is divided by the priority for each row (Mu & Pereyra-Rojas 2017:13–14). Random consistency (RC / Eqn 5) is dependent on the number of alternatives (in the example of Ahammed and Azeem there are three). The RC increases proportionally with the number of alternatives (Ahammed & Azeem 2013:9). For example, for n = 3 the value for RC = 0.58, and for n = 5 the value for RC = 1.12 (Ahammed & Azeem 2013:9).
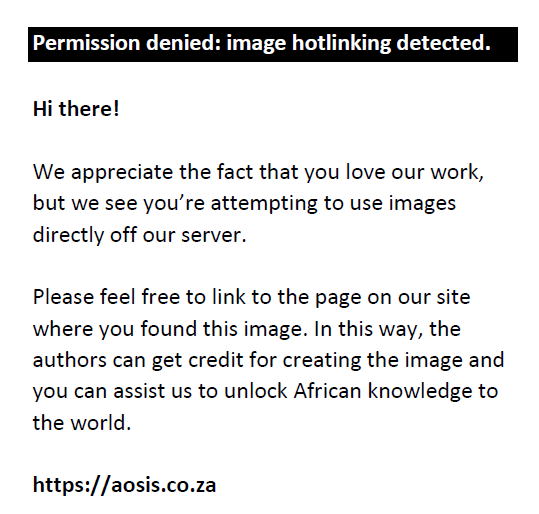
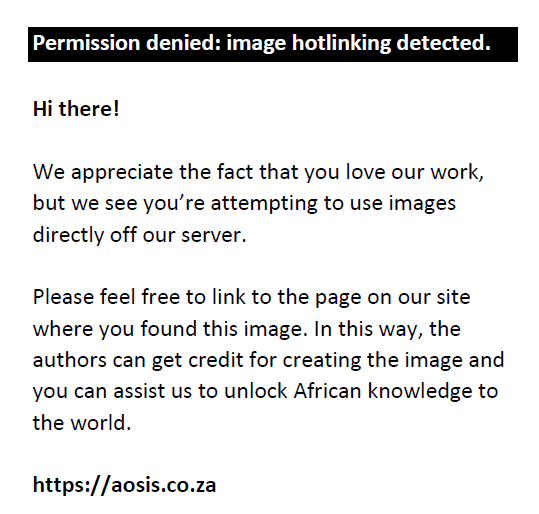
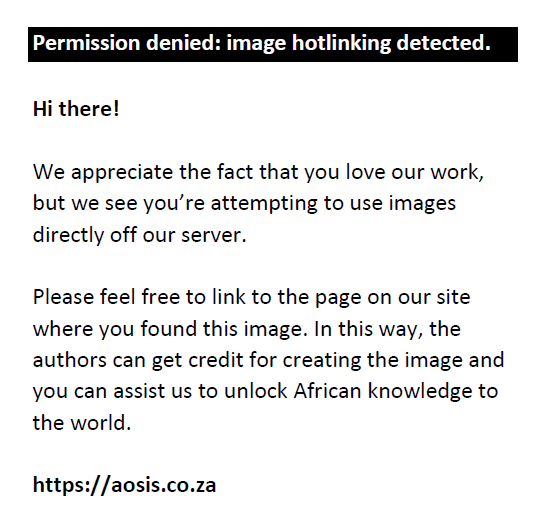
It is acceptable to have CR ≤ 0.10 and to review (or reject) selections where CR > 0.10 (Ahammed & Azeem 2013:8). However, in practice it is not uncommon to accept CR > 0.10 and CR < 0.20, which is still considered reasonable (Pauer et al. 2016:5). After review, the final decision matrix that represents the basis for relative weights for the alternatives can be calculated. The calculation of the relative weights for the illustrated example is also presented (Ahammed & Azeem 2013:9), where:
- A − Cx= Alternatives for selection criteria cost (x)
- A − Cy= Alternatives for selection criteria demand (y)
- A − Cz= Alternatives for selection criteria availability (z)
In the example of Ahammed and Azeem, the relative weights for the alternatives were calculated by multiplying the decision matrix with the weighting of each selection criteria (Eqn 6 & 7):
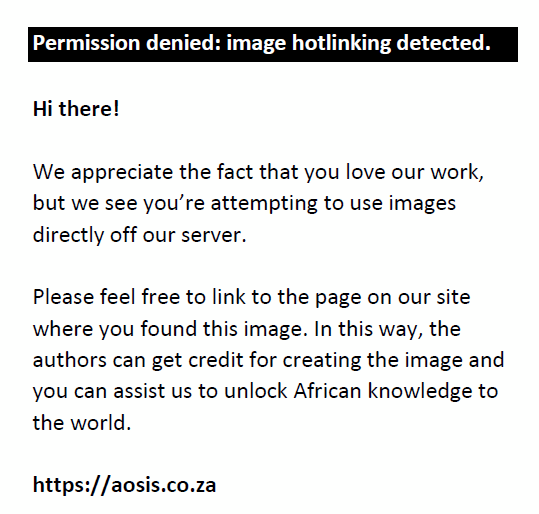
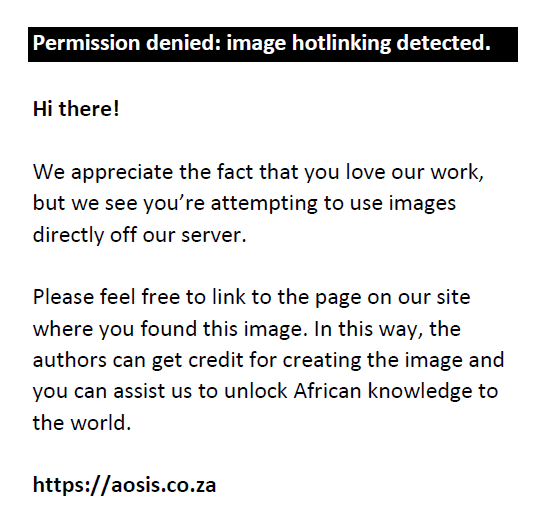
In the example from Ahammed and Azeem (the solar power system), 30 Wp is calculated to be the most desirable option because of the relative importance of low price (CP = 0.5940). The same principle can be applied to the BSC where the relative importance of each of the perspectives and KPIs can be related to the underlying weighting for each BSC metric.
The findings of this research could provide manufacturing SME managers and owners with little or no accounting knowledge, with a generic BSC template that could serve as a management tool. Furthermore, it would contribute to existing literature by providing a framework to develop generic BSCs in any context. The research method followed is explained next, followed by the results of the Delphi study, the findings and the conclusion.
Research method and design
Methodology
The research was conducted in a mixed-method research paradigm using a Delphi study over a period of 10 months. According to Bryman (2016:635), a mixed-method approach uses the principles of both quantitative and qualitative research techniques. In this study, qualitative data (open-ended responses), as well as quantitative data (close-ended responses) were collected. Furthermore, the data were analysed using qualitative techniques (thematic coding) and quantitative techniques such as AHP and descriptive statistics. The research therefore adopted a pragmatic stance that included significant interpretivist interaction with Delphi panel members. A comprehensive literature review was first conducted. Purposive, heterogeneous sampling was used to identify the 27 panel members for the Delphi study. The 27 panel members represented cost accounting experts from academia and practice, representing a range of industries. Holloway and Galvin (2016:146) describe heterogeneous sampling as when individual members can be differentiated from each other by a distinct characteristic. In this case, the panel members could be divided into two distinct groups: cost accounting academics and cost accounting industry experts. The sample of participants was sourced from previously established networks, social networks and universities. The study used descriptive statistics, content analysis and the AHP to analyse the feedback from the panel members.
Validity and reliability
The concepts used for the data analysis such as descriptive statistics, content analysis and AHP were adequately understood by the authors as demonstrated in the development of the generic BSC to ensure the validity of the statistical analysis. To ensure the reliability of the content analysis in Round 2, the final analysis was reviewed by an independent accounting expert. Furthermore, the Microsoft Excel for Mac template, developed for the AHP analysis conducted after the conclusion of Round 3, was reviewed and confirmed as applicable by an independent academic with expertise on the concepts of AHP. Causal reliability was demonstrated by the literature review that illustrated that AHP can be effectively used to develop the BSC because of its hierarchical structure. External validity is addressed by the use of a heterogeneous expert panel from different industries, as well as academics. As a result, the development of the research instruments (surveys) was based on past literature and the feedback from a Delphi panel made up of people who can be considered experts in the field of cost accounting.
Development of the generic balanced scorecard
The surveys used as part of the Delphi study were conducted on an online survey platform (SurveyMonkey) over three rounds, after which it was possible to present the generic BSC for manufacturing SMEs. The development of the generic BSC commenced with the identification of the 27 expert panel members and proceeded with an iterative process of research instrument design, surveys and data analysis. The process concluded with the development of the generic BSC after the completion of Round 3. The development of the generic BSC is outlined in Figure 2.
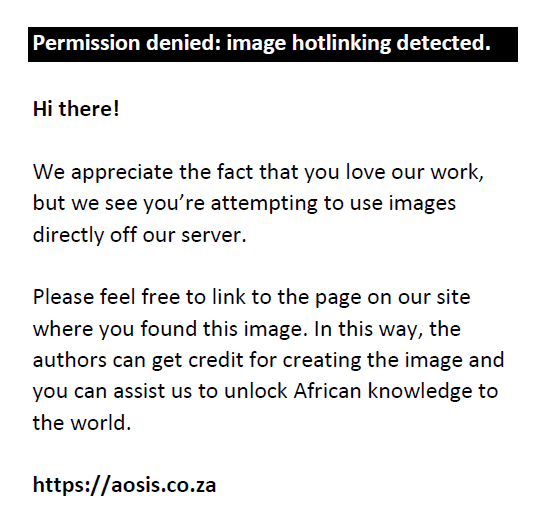 |
FIGURE 2: Delphi process used to develop the generic balanced scorecard. |
|
Delphi study Round 1: Measurability of critical success factors within activities
As mentioned previously, the generic CSFs and activities for manufacturing SMEs as identified in literature were adopted as a starting point for the Delphi study (Figure 3). As depicted in Figure 3, the five generic CSFs were allocated to each of the four BSC perspectives for a total of 20 potential KPIs. However, if the six generic manufacturing SME activities are considered for the generic BSC and there is a possibility to assign a KPI for each BSC perspective, generic CSF and activity combination, the total number of KPIs can potentially total 120 (excluding KPIs not reserved for any activity). This number of KPIs would not be practical for a manufacturing SME and it was therefore decided to use Round 1 to eliminate the number of KPIs that could be measured within activities.
 |
FIGURE 3: Generic critical success factors for manufacturing small and medium enterprises. |
|
Expert panel members were asked to assign the degree of measurability of each perspective, CSF and activity combination using a Likert-type scale (1 = not measurable at all; 2 = slightly measureable; 3 = moderately measurable; 4 = fully measurable; and 5 = extremely measurable). Of the 27 panel members, 23 participated in the survey (85% response rate). The response rate was considered more than sufficient for the purpose of the study. To establish which activity metric combination should be included in the generic BSC it was decided to only consider responses of 4 (fully measurable) or 5 (extremely measurable) as representing consensus. Activity metric combinations with an overall consensus of less than 70% were excluded from the generic BSC. An additional qualification criterion (mean ≥ 4) was included. Therefore, a combination of perspective and measurement category was only considered if it satisfied both the criteria of consensus and mean. An overview of the analysis and the results is provided in Appendix 1.
Delphi study Round 2: Identifying specific key performance indicators
In Round 1 expert panel members were requested to select the degree of measurability for each perspective–KPI category–activity combination. On conclusion of the survey, the number of potential measurement categories at activity level was reduced from 120 to 9. The next step was to consider the specific KPIs that can be measured in the generic BSC. Although the purpose of the study was to develop a generic BSC, it was considered that some flexibility must be available for manufacturing SME entrepreneurs and managers to adapt the BSC to their specific circumstances. The survey was divided into the four perspectives with the five KPI categories assigned to each metric. Each perspective was further divided into two sections: factory-level metrics and activity-level metrics. Expert panel members were requested to identify at least one KPI per metric category.
Round 2 had a significantly lower response rate than Round 1. Only 11 of the 27 panel members that were approached responded (41% response rate). Despite the lower response rate, a total number of 104 KPIs could be identified from the responses of the panel members. Because of the nature of the open-ended responses, the individual responses from the panel members were analysed using content analysis (available on request). An additional column was created where each specific KPI identified from the responses was entered. A further 11 columns were then created to capture responses. A corresponding response from a respondent was entered next to a specific KPI where it is found to be similar or identical. Any response was considered if it was judged to be specific and measurable; for example, ‘quality control report’ could not be included as it cannot be measured. The 104 specific KPIs identified from the content analysis were divided into 16 homogeneous metric groups representing generic KPIs (Appendices 2 and 3). The purpose of the generic KPIs was to provide the option of selecting appropriate specific KPIs to owners and managers of manufacturing SMEs.
Delphi study Round 3: Rating the relative importance of balanced scorecard elements
In Round 2, expert panel members were requested to identify specific and measurable KPIs that were grouped into 32 homogeneous metric groups (16 general KPIs identified from Round 2 on factory and activity levels). The purpose of Round 3 was to further reduce the number of general KPIs and to determine the number of KPIs that should be included in the BSC for the manufacturing SME. The first question on the survey requested panel members to identify the number of KPIs to be included in the BSC for manufacturing SMEs. A drop-down list was used for this purpose. The remainder of the survey used pairwise comparisons to enable panel members to decide on the relative importance of BSC perspectives and general KPIs within the categories (factory or activity level). The pairwise comparisons were scaled using the following terms: 1 – equal importance; 3 – moderately more important; 5 – essentially more important; 7 – very strong importance (over another KPI); and 9 – absolute importance (over another KPI). Of the 27 panel members, a total of 17 completed the survey (63% response rate). The improved response rate from Round 2 can be attributed to the use of questions requiring closed-ended responses in Round 3 instead of the open-ended responses used in Round 2.
Developing the generic balanced scorecard
The generic BSC for manufacturing SMEs was developed using the following general steps:
- Step 1: Calculate the number of KPIs suitable for manufacturing SMEs from the responses received from the panel members.
- Step 2: Apply the AHP to calculate the relative weights of the BSC perspectives, categories and KPIs.
- Step 3: Allocate the correct number of KPIs to each perspective and category.
- Step 4: Rank each KPI by its overall weighting, as well as the maximum weighting achieved for a single segment (combination of perspective and category).
- Step 5: Calculate a combined ranking for the overall weighting and the maximum segment weighting.
- Step 6: Assign the KPIs to the BSC individually by starting with the highest ranking KPI and assigning it to the segment with the highest relative weight that is still available.
To calculate the number of general KPIs, the median (M = 12) was deemed appropriate because of the high standard deviation (s = 7.09) in the sample. The mean (x̅ = 14.76) was affected by three outliers as extremely high values (30; 25; 25). The total number of general KPIs to be included in the generic BSC for manufacturing SMEs is therefore 12.
The next step was to use the AHP to calculate the weighting for the BSC perspectives and categories (factory or activity). At first, the data collected from the decision-makers were organised in a comparison matrix and a total was calculated for each column (Table 2 upper section). The relative weight for element in the comparison matrix was then calculated for the normalised matrix by dividing each element by the relevant total from the comparison matrix (Table 2 lower section). This process was repeated for each decision-maker as a basis for the priority weight calculations.
TABLE 2: Comparison and normalised matrix. |
The priority weights for each criterion were calculated from the average of each row in the normalised matrix. For example, the priority weight for the financial perspective was calculated as (0.192 + 0.318 + 0.313 + 0.125) / 4 = 0.237. To calculate λmax, the priority weights are transferred to a weighted comparison matrix where it is multiplied with the comparison matrix (Table 3). The row total is then divided by the average to determine a consistency measure that is used to calculate λmax. Ultimately the average value of four consistency measures were used. For example, the consistency value for customer is calculated as 0.331 (priority weight) divided into 1.407 (sum of row weighted comparison) = 4.25. The 4.30 that was calculated for λmax could also be calculated by using the matrix product function (MMULT) in Microsoft Excel for Mac using the parameters (comparison row and priority column) and then dividing by the average.
TABLE 3: Weighted comparison matrix with λmax. |
The next step was to calculate the consistency index and consistency ratio using the formulae as identified in literature (Eqn 8 – 10):
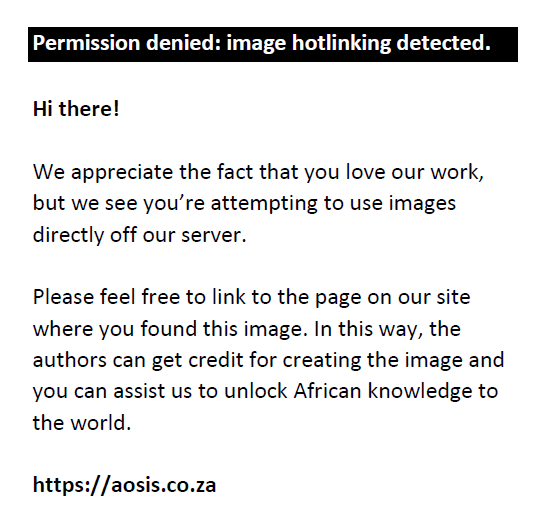
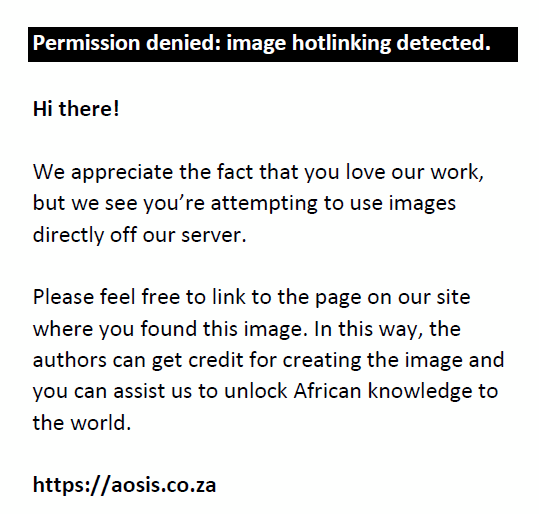
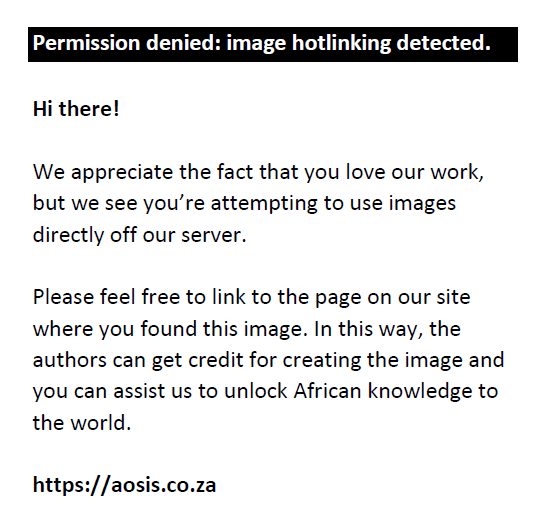
It was established from literature that selections with CR < 0.10 should be accepted but that CR < 0.20 can also be considered as appropriate in some cases. It was calculated from the AHP calculation that if different CR criteria are applied (between < 0.10 and < 0.20) that the results only differ marginally across a range of criteria (Table 4). The overall results were calculated by using the arithmetic mean for qualifying responses. The financial and customer perspective were preferred over the internal perspective ranging from 63% combined for CR < 0.20 to 72% combined for CR < 0.10 as qualifying criteria. The number of qualifying responses increased from 6 to 9 (out of 15 selections) if CR inclusion rate is relaxed from < 0.100 to < 0.125 and only increased again by another 2 when CR < 0.20 is applied. It appears that adequate consistent and sufficient data collection may be applicable if CR is set between < 0.125 and < 0.175. Based on this premise and the relative consistency of the results across the different inclusion criteria, the remainder of the AHP discussion will be based on the result from CR < 0.150.
TABLE 4: Perspective overall weighting for different acceptance criteria of consistency ratio. |
From the AHP calculation based on CR < 0.15, it was possible to calculate the overall weights and segment weights for each general KPI. The next step was to assign the 12 generic KPIs to each perspective and segment (factory and activity level). It was already established that the financial and customer perspectives (of relative equal stature) are preferred by decision-makers in a ratio of approximately 2:1. Therefore, a total number of 8 general KPIs (out of 12) were assigned to the financial and customer perspectives in equal measure. The remaining four general KPIs were assigned in equal measure to each of the four segments for the internal and learning perspectives. Because only one general KPI was available for the learning activity-level segment and it was considered more important than the other three segments, it was considered the only appropriate strategy. The preceding approach is outlined in Figure 4.
 |
FIGURE 4: Number of key performance indicators for balanced scorecard with CR < 0.15. †Learning and growth activity level only has one generic key performance indicator available. |
|
The next step was to divide the eight generic KPIs assigned to the financial and customer perspectives to the four activity segments (Figure 5). The expert panel preferred 66.06% of KPIs in the financial perspective to be assigned to the activity level. Three generic KPIs were assigned to the activity level and the remaining KPI were assigned to the factory level. The result for the customer perspective was closer and the KPIs were therefore equally assigned to the factory level and the activity level. The generic BSC were therefore established to include four financial perspective KPIs (one factory and three activity), four customer perspective KPIs (two each for factory and activity), two internal perspective KPIs (one each for factory and activity) and two learning perspective KPIs (one each for factory and activity).
 |
FIGURE 5: Number of key performance indicators to financial and customer perspectives. |
|
To establish a priority ranking for the general KPIs it was considered that an important general KPI within a lowly weighted perspective might be eliminated by a general KPI within a highly weighted perspective. The general KPIs were ranked by means of a combination ranking, which is a combination of overall ranking and highest segment weighting. The next step was to assign the general KPIs individually to the BSC in order of combined ranking by its highest available segment weight (Table 5). In this case, the first general KPI that could be assigned is manufacturing performance. It was assigned to the learning and growth perspective in the production activity, with a segment weighting of 1.000 as it was the only element in this context. Using this approach, it was possible to assign 12 general KPIs (out of 16) to the generic BSC. Three generic KPIs in the learning and growth perspective on factory level (employee education, information technology and employee satisfaction) would not be assigned as only one general KPI was required in the segment, which in this case was production technology; it had an average ranking of eight, and an overall ranking of seven. Furthermore, the general KPI cost of obtaining funds was ranked last (16) in all measures and was therefore not included.
TABLE 5: Ranking of general key performance indicators for the generic balanced scorecard with consistency ratio < 0.15. |
Ethical consideration
Ethical clearance was obtained prior to the commencement of the research from the Nelson Mandela University (Ref: H-15-BES-ACC-020). The cost accounting experts were supplied with an information booklet outlining the purpose and scope of the study. A section where the participant was required to provide informed consent was provided for at the beginning of each survey. The surveys did not require the participants to divulge their names or any personal details. Furthermore, the Internet Protocol (IP) addresses of the participants were not tracked during the process of conducting the surveys to maintain their anonymity.
Results
In Round 1, the expert panel only assigned nine perspective and generic CSF combinations to activities constituting a small proportion (7.5%) of all combinations (Table 6). In the financial perspective, revenue growth was paired with the sales and distribution function, which is consistent with the functionality of this activity (Jespersen & Skjøtt-Larsen 2005:138). The purchasing function was associated with cost savings by expert panel members. It confirms the importance of the purchasing function for a manufacturing SME to ensure that profit margins are maximised (Hung et al. 2015:199). The customer perspective and the internal perspective each found three activities associated with performance measurement. Service delivery was associated with the customer service function, which is described as a core activity in the SCM function (Jespersen & Skjøtt-Larsen 2005:18). Delivering a quality product was considered significant in the context of the production and product development function. Although quality is relevant across an organisation (Benzing et al. 2009:63; Ghosh et al. 2001:211), the expert panel may have wanted to focus scarce resources on the primary activities. Cost reduction (as an internal activity, as opposed to monetary result) was considered important in the production activity as well as in the purchasing function. Furthermore, on-time delivery was considered relevant for the sales and distribution function. In the learning and growth perspective, only production measure could be associated with an activity. In this case, it was associated with production and product development.
TABLE 6: Combinations selected in Round 1. |
The expert panel identified 104 specific KPIs for manufacturing SMEs in Round 2. It was necessary to group the specific KPIs into 16 categories (general KPIs) for further evaluation (Appendix 2 and 3). It was found that the expert panel identified specific KPIs that were fundamentally similar but could be used in different settings. It is likely that various manufacturing SMEs may have slightly different preferences with regards to the selection of specific KPIs. Therefore, it was established that a generic BSC should have sufficient flexibility within a formal structure. It was found that the BSC implementation may fail if a structured approach is not followed for the design of the BSC (Andersen et al. 2001:6; Fernandes et al. 2006:627).
The generic BSC (Figure 6) conforms to this belief by only including 12 metrics for manufacturing SMEs’ performance measurement, yet it still allows the entrepreneurs the flexibility of selecting appropriate KPIs for their industry. It is suggested that the effectiveness of the generic BSC for manufacturing SMEs should be empirically tested to determine the suitability in different manufacturing industries. It is possible that an empirical study of this nature could adapt and improve the generic BSC even further. Furthermore, it is recommended that a similar study be conducted with alternative development techniques not used in this research.
 |
FIGURE 6: The generic balanced scorecard for small and medium manufacturing enterprises in South Africa. KPI, key performance indicator. |
|
The results from the AHP calculation enabled the 12 general KPIs to be included in the generic BSC for manufacturing SMEs to be allocated according to the preference from the expert panel. In Round 3 it was found that the financial and customer perspectives were considered more important to the expert panel compared to the internal and learning perspectives (Table 4).
The rationale behind the generic BSC is that owners and entrepreneurs of manufacturing SMEs select a specific KPI relevant to their organisation from the data collected in Round 2, for example, cash flow is required to be measured once in the generic BSC. However, manufacturing SMEs can also select one of the following KPIs, namely cash availability, cash conversion cycle, inventory value, creditors versus debtor’s days, number of days with positive cash flow, cash flow from operations or availability of overdraft facility. The generic BSC is presented in Figure 6, listing the specific KPIs that manufacturing SMEs can select for each of the 12 generic KPIs.
Conclusion
The primary purpose of the research was to develop and present a generic BSC for manufacturing SMEs in South Africa. The basis for the development of the generic BSC was the generic CSFs identified in the literature review. The generic CSFs were classified in broadly the same categories as the four BSC perspectives. Furthermore, the generic BSC was developed to allow flexibility for manufacturing SMEs that may have slightly different requirements. This was achieved by including the 12 general KPIs in the generic BSC that each includes numerous specific KPIs, as identified by the expert panel.
In general, the findings from the expert panel during development of the generic BSC confirmed the premise that the BSC for manufacturing SMEs should be uncomplicated and easy to use. The ability to adapt the generic BSC to the needs of the manufacturing SMEs, by incorporating sufficient flexibility, represents a management tool that could be adapted to many settings. Furthermore, using cost accounting experts to develop the generic BSC ensures that the final instrument has a sound development basis and can be reliably used in practice. The researchers are therefore confident that an appropriate performance measurement system has been developed for manufacturing SMEs, representing a significant contribution to existing literature.
Recommendations and suggestions for future research
It is recommended that owners and management of manufacturing SMEs adapt the generic BSC with due consideration of the specific KPIs applicable to their organisations. It is also advised that easily measurable KPIs be selected that do not require additional resources. Furthermore, it is suggested that future research attempt to measure the suitability of the generic BSC by means of an implementation case study. It is proposed that a case study be performed for a period of time and the suitability of integrating with costing systems be established.
Acknowledgements
The authors are grateful for the contributions from all the cost accounting experts who participated in this research. Funding was received from a post-graduate bursary for PhD studies from Nelson Mandela University.
Competing interests
The authors declare that they have no financial or personal relationship(s) that may have inappropriately influenced them in writing this article. The views expressed in the submitted article are the authors’ own and do not necessarily reflect the official position of the listed institutions.
Authors’ contribution
A.R. constructed the article from the research conducted during the PhD study; H.F. was responsible for academic insight and review; and L.E. was responsible for academic insight and review.
References
Afonso, H. & Cabrita, M.D.R., 2015, ‘Developing a lean supply chain performance framework in a SME: A perspective based on the balanced scorecard’, Procedia Engineering 131(1), 270–279. https://doi.org/10.1016/j.proeng.2015.12.389
Ahammed, F. & Azeem, A., 2013, ‘Selection of the most appropriate package of solar home system using analytic hierarchy process model in rural areas of Bangladesh’, Renewable Energy 55(1), 6–11. https://doi.org/10.1016/j.renene.2012.12.020
Andersen, H., Cobbold, I. & Lawrie, G., 2001, ‘Balanced scorecard implementation in SME’s: Reflection on literature and practice’, 2CG conference paper, 4th SME International Conference, May 2001, Allborg University, Denmark.
Antoncic, J.A. & Antoncic, B., 2011, ‘Employee satisfaction, intrapreneurship and firm growth: A model’, Industrial Management & Data Systems 111(4), 589–607. https://doi.org/10.1108/02635571111133560
Asare, R., Akuffobea, M., Quaye, W. & Atta-Antwi, K., 2015, ‘Characteristics of micro, small and medium enterprises in Ghana: Gender and implications for economic growth’, African Journal of Science, Technology, Innovation and Development 7(1), 26–35. https://doi.org/10.1080/20421338.2014.979651
Avcikurt, C., Altay, H. & Ilban, M.O., 2011, ‘Critical success factors for small hotel businesses in Turkey: An exploratory study’, Cornell Hospitality Quarterly 52(2), 153–164. https://doi.org/10.1177/1938965509341288
Ayvaz, E. & Pehlivanl, D, 2011, ‘The use of time driven activity based costing and analytic hierarchy process method in the balanced scorecard implementation’, International Journal of Business and Management 6(3), 146–158. https://doi.org/10.5539/ijbm.v6n3p146
Belekoukias, I., Garza-Reyes, J.A. & Kumar, V., 2014, ‘The impact of lean methods and tools on the operational performance of manufacturing organisations’, International Journal of Production Research 52(18), 5346–5366. https://doi.org/10.1080/00207543.2014.903348
Benzing, C., Chu, H.M. & Kara, O., 2009, ‘Entrepreneurs in Turkey: A factor analysis of motivations, success factors, and problems’, Journal of Small Business Management 47(18), 58–91. https://doi.org/10.1111/j.1540-627X.2008.00262.x
Bhagwat, R. & Sharma, M.K., 2007, ‘Performance measurement of supply chain management: A balanced scorecard approach’, Computers & Industrial Engineering 53(1), 43–62. https://doi.org/10.1016/j.cie.2007.04.001
Brink, A., Cant, M. & Ligthelm, A., 2003, ‘Problems experienced by small businesses in South Africa’, Small Enterprise Association of Australia and New Zealand 16th Annual Conference, Ballarat, Australia, 28 Sept. – 01 Oct., 2003, pp. 1–20.
Bryman, A., 2016, Social research methods, 5th edn., Oxford, New York.
Bureau for Economic Research, 2016, The small, medium and micro enterprise sector of South Africa commissioned by the Small Enterprise Development Agency, viewed 22 May 2018, from http://www.seda.org.za/Publications/Publications/The%20Small,%20Medium%20and%20Micro%20Enterprise%20Sector%20of%20South%20Africa%20Commissioned%20by%20Seda.pdf
Callado, A.A. & Jack, L., 2015, ‘Balanced scorecard metrics and specific supply chain roles’, International Journal of Productivity and Performance Management 64(2), 288–300. https://doi.org/10.1108/IJPPM-05-2014-0071
Charantimath, P.M., 2011, Total quality management, 2nd edn., Pearson Education, New Delhi.
Dhurup, M. & Makhita, K.M., 2014, ‘Reflections on the constraints in entrepreneurial development among emerging survivalist and micro craft enterprises in Gauteng’, Journal of Contemporary Management 11(1), 230–250.
Engle, R.L., 2005, ‘Global marketing management scorecard: A tale of two multinational companies’, Problems and Perspectives in Management 3(1), 128–136.
Fernandes, K., Raja, V. & Whalley, A., 2006, ‘Lessons from implementing the balanced scorecard in a small and medium size manufacturing organisation’, Technovation 26(1), 623–634. https://doi.org/10.1016/j.technovation.2005.03.006
Ghosh, B.C., Liang, T.W., Meng, T.T. & Chan, B., 2001, ‘The key success factors, distinctive capabilities, and strategic thrusts of Top SMEs in Singapore’, Journal of Business Research 51(1), 209–221. https://doi.org/10.1016/S0148-2963(99)00047-8
Gono, S., Harindranath, G. & Özcan, G.B., 2014, ‘Understanding the impact of ICT adoption and use in South African manufacturing and logistics SMEs: A firm, market and regulatory (FMR) context perspective’, in Proceedings of ISBE 2014 Conference, Manchester, England, 05–06 November, 2014, pp. 1–20.
Gunasekaran, A., Patel, C. & McGaughey, R.E., 2004, ‘A framework for supply chain performance measurement’, Internal Journal of Production Economics 87(1), 333–347. https://doi.org/10.1016/j.ijpe.2003.08.003
Hasson, F., Keeney, S. & McKenna, H., 2000, ‘Research guidelines for the Delphi Survey technique’, Journal of Advanced Nursing 32(4), 1008–1015.
Holloway, I. & Galvin, K., 2016, Qualitative research in nursing and healthcare, 4th edn., John Wiley & Sons, Chichester.
Hung, S.C., Hung, S.W. & Lin, M.J., 2015, ‘Are alliances a panacea for SMEs? The achievement of competitive priorities and firm performance’, Total Quality Management & Business Excellence 26(1–2), 190–202. https://doi.org/10.1080/14783363.2014.927133
Ingle, M., 2014, ‘An analysis of factors impeding SMME performance in South Africa’, Africa Insight 44(2), 37–50.
Jeon, H. & Choi, B., 2012, ‘The relationship between employee and customer satisfaction’, Journal of Services Marketing 26(5), 332–341. https://doi.org/10.1108/08876041211245236
Jespersen, B.D. & Skjøtt-Larsen, T., 2005, Supply chain management: In theory and practice, Copenhagen Business School Press, Copenhagen.
Kahn, K.B., 2015, Product planning essentials, 2nd edn., Routledge, New York.
Kaplan, R.S. & Norton, D.S., 1992, ‘The balanced scorecard: Measures that drive performance’, Harvard Business Review Jan–Feb, 71–79.
Kelly, M., Mokyr, J. & O’Grada, C., 2014, ‘Precocious Albion: A new interpretation of the British industrial revolution’, Annual Review of Economics 6(1), 363–389. https://doi.org/10.1146/annurev-economics-080213-041042
Khan, A.J. & Tidke, D.J., 2013, ‘Designing facilities layout for small and medium enterprises’, International Journal of Engineering Research and General Science 1(2), n.p.
Kirsten, E., Vermaak, F. & Wolmarans, H., 2015, ‘Performance measurement in small and medium enterprises: South African accountants view’, Journal of Economic and Financial Sciences 8(1), 13–34.
Kumar, V., Verma, P., Onkar, S.P.S. & Katiyar, J., 2016, Facility and process layout analysis of an SME using simulation: A case study of a manufacturing company, viewed 22 May 2018, from http://ieomsociety.org/ieom_2016/pdfs/354.pdf
Lin, H.F., 2015, ‘Linking knowledge management orientation to balanced scorecard outcomes’, Journal of Knowledge Management 19(6), 1224–1249. https://doi.org/10.1108/JKM-04-2015-0132
Mehralian, G., Nazari, J.A., Nooriparto, G. & Rasekh, H.R., 2017, ‘TQM and organizational performance using the balanced scorecard approach’, International Journal of Productivity and Performance Management 66(1), 111–125. https://doi.org/10.1108/IJPPM-08-2015-0114
Mendis, G.H. & Ganga, G.M., 2013, ‘Predicting success in product development: The application of principal component analysis to categorical data and binomial logistic regression’, Journal of Technology Management & Innovation 8(3), 83–97. https://doi.org/10.4067/S0718-27242013000400008
Mokhtar, S.S., Yusoff, R.Z. & Ahmad, A., 2014, ‘Key elements of market orientation on Malaysian SMEs’ performance’, International Journal of Business and Society 15(1), 49–64.
Mokhtar, S.S., Yusoff, R.Z. & Arshad, R., 2009, ‘Market orientation critical success factors of Malaysian manufacturers and its impact on financial performance’, International Journal of Marketing Studies 1(1), 77–84. https://doi.org/10.5539/ijms.v1n1p77
Moyo, T., 2003, ‘Critical success factors for microenterprise development in Africa: An overview’, Transformation 20(3), 166–170. https://doi.org/10.1177/026537880302000310
Mu, E. & Pereyra-Rojas, M., 2017, ‘Understanding the analytical hierarchy process’, Practical decision making, Springer Briefs in Operational Research, pp. 7–22, Springer, Cham. https://doi.org/10.1007/978-3-319-33861-3_2
Ng, H. & Kee, D., 2012, ‘The issues and development of critical success factors for the SME success in developing countries’, International Business Management 6(6), 680–691. https://doi.org/10.3923/ibm.2012.680.691
Nkosi, E., Bounds, M. & Goldman, G., 2013, ‘Skills required for the management of black-owned small enterprises in Soweto’, Acta Commercii 13(1), 1–10. https://doi.org/10.4102/ac.v13i1.186
Nuntsu, N., Tassiopoulos, D. & Haydam, N., 2004, ‘The bed and breakfast market of Buffalo City (BC), South Africa: Present status, constraints and success factors’, Tourism Management 25(4), 515–522. https://doi.org/10.1016/S0261-5177(03)00125-0
Obi, S.C., 2013, Introduction to manufacturing systems, 1st edn., Authorhouse, Bloomington, IN.
Okongwu, U., Brulhart, F. & Moncef, B., 2015, ‘Causal linkages between supply chain management practices and performance: A balanced scorecard strategy map perspective’, Journal of Manufacturing Technology Management 26(5), 678–702. https://doi.org/10.1108/JMTM-01-2013-0002
Okpara, J.O. & Kabongo, J.D., 2009, ‘An empirical evaluation of barriers hindering the growth of Small and Medium Sized Enterprises (SMEs) in a developing economy’, Journal of Emerging Trends in Economics and Management Sciences 5(7), 1–6.
Okpara, J.O. & Wynn, P, 2007, ‘Determinants of small business growth constraints in a sub-Saharan African economy’, SAM Advanced Management Journal Spring, 72(2), 24–35.
Olawale, F. & Garwe, D., 2010, ‘Obstacles to the growth of new SME’s in South Africa: A principal component analysis approach’, African Journal of Business Management 4(5), 729–738.
Onaolapo, A.A. & Oladejo, M.O., 2011, ‘Effectiveness of millennium development goal programmes on entrepreneurial development: An appraisal of the Nigerian experience’, Journal of Emerging Trends in Economics and Management Sciences 2(4), 310–319.
Pauer, F., Schmidt, K., Babac, A., Damm, K., Frank, M. & von der Schulenburg, J.-M.G., 2016, ‘Comparison of different approaches applied in analytic hierarchy process – An example of information needs of patients with rare diseases’, BMC Medical Informatics and Decision Making 16(1), 117, viewed 22 May 2018, from https://www.ncbi.nlm.nih.gov/pmc/articles/PMC5016921/
Rajput, R.K., 2007, A textbook of manufacturing technology: Manufacturing processes, Laxmi, New Delhi.
Remenyi, D., 2013, Field methods for academic research, 3rd edn., Academic Conferences and Publishing, London.
Rockart, J., 1979, ‘Chief executives define their own data needs’, Harvard Business Review 57(2), 81–93.
Saaty, T.L., 2008, ‘Decision making with the analytic hierarchy process’, International Journal of Services Sciences 1(1), 83–98. https://doi.org/10.1504/IJSSCI.2008.017590
Santos-Requejo, L. & González-Benito, O., 2000, ‘Economic success factors in Spanish small retail businesses. An analysis based on sector-relative definitions’, Small Business Economics 15(3), 209–222. https://doi.org/10.1023/A:1008109512688
Sebone, M.F. & Barry, M.L., 2009, ‘Toward determining critical success factors for SME electrical contractors in the construction industry in South Africa’, South African Journal of Industrial Engineering 20(1), 185–195.
Sesep, C., 2016, ‘Growth barriers facing South African manufacturing small and medium enterprises’, Master of Commerce dissertation, Faculty of Management, University of Johannesburg, viewed 22 May 2018, from https://ujcontent.uj.ac.za/vital/access/manager/Repository/uj:22829.
Shelton, K. & Creghan, K.A., 2015, ‘Demystifying the Delphi method’, in V.C.X. Wang, Handbook of Research on Scholarly Publishing and Research Methods, pp. 375–396, IGI Global, Hershey, PA.
Statistics South Africa, 2017, ‘Quarterly financial statistics (QFS): Statistical release, viewed 22 May 2018, from http://www.statssa.gov.za/publications/P0044/P0044March2017.pdf
Temtime, Z.T. & Pansiri, J., 2004, ‘Small business critical success/failure factors in developing economies: Some evidence from Botswana’, American Journal of Applied Science 1(1), 18–25. https://doi.org/10.3844/ajassp.2004.18.25
Varma, S., Wadhwa, S. & Deshmukh, S.G., 2008, ‘Evaluating petroleum supply chain performance: Application of analytical hierarchy process to balanced scorecard’, Asia Pacific Journal of Marketing and Logistics 20(3), 343–356. https://doi.org/10.1108/13555850810890093
Yusuf, A., 1995, ‘Critical success factors for small business: Perceptions of South Pacific entrepreneurs’, Journal of Small Business Management April, 33, 68–73.
Appendix 1
Appendix 2
TABLE 1-A2: Specific metrics identified in Round 2 (Part 1). |
Appendix 3
TABLE 1-A3: Specific metrics identified in Round 2 (Part 2). |
|